- Published on
Figure Is Introducing Learned Natural Walking
- Authors
- Name
- The Editorial Team
- @humanoidsdaily
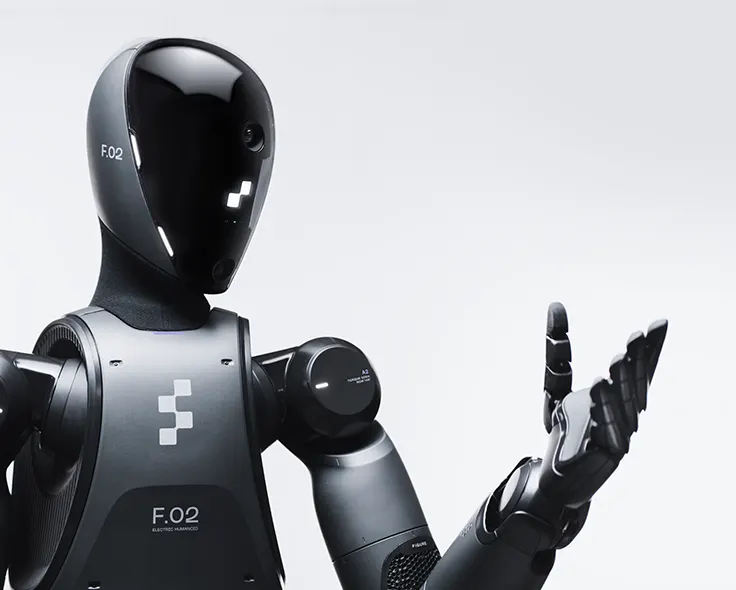
Bridging the Sim-to-Real Gap: A New Era in Humanoid Robotics
The landscape of humanoid robotics is undergoing rapid change, propelled by advancements in reinforcement learning (RL). A groundbreaking end-to-end neural network developed by Figure is setting new standards in humanoid locomotion, utilizing RL to achieve human-like walking in their Figure 02 robot. This innovation promises significant strides, yet it also raises important questions about the scalability and robustness of these technologies.
Leveraging Simulation for Human-Like Movement
At the core of Figure's approach is the application of RL within high-fidelity physics simulations. By running thousands of virtual robots under varied conditions, the team effectively simulates years' worth of data within mere hours. This method allows the RL controller to develop a robust, transferable policy for walking, bringing human-like gait, heel-strike, and arm-leg synchronization into the robotic realm.
The reliance on simulations addresses a critical bottleneck: real-world testing can be time-consuming and resource-intensive. By contrast, simulations offer a cost-effective, rapid alternative, albeit with the recognition that they are approximations of reality. Notably, Figure's simulations incorporate diverse scenarios, from varying terrains to mechanical disturbances, ensuring a well-rounded training process.
Sim-to-Real Transfer: Addressing the Gap
Transferring learned behavior from simulations to real-world robots—known as the "sim-to-real gap"—presents notable challenges. Figure tackles this through domain randomization, wherein physical parameters are varied extensively in simulations, helping the policy to generalize better when tested on actual hardware. Moreover, the use of high-frequency torque feedback enhances the real-world application by accounting for discrepancies in actuator dynamics.
This methodology has shown promising results, evidenced by consistent human-like walking across a fleet of Figure 02 robots. The potential for zero-shot transfer without additional tuning underscores the robustness and adaptability of the developed policy.
Engineering Challenges and Future Prospects
Despite the encouraging results, Figure acknowledges the inherent complexities in achieving truly human-like locomotion. While the robots emulate human gait closely, further refinement is necessary to capture subtler elements of human movement. Moreover, as the fleet size scales, ensuring consistency and efficiency without manual intervention will be paramount.
The scalability of this technology to thousands or even millions of humanoid robots presents both an opportunity and a challenge. Successfully deploying such systems could revolutionize sectors reliant on humanoid robotics, including logistics, healthcare, and domestic assistance.
Conclusion
Figure's work represents a significant leap forward in humanoid robotics, illustrating the potential of RL and high-fidelity simulations in solving complex locomotion problems. Yet, several challenges remain—especially in terms of scaling and optimizing behavior in highly dynamic environments.